Bias in the machine: How can we address gender bias in AI?
At the Raspberry Pi Foundation, we’ve been thinking about questions relating to artificial intelligence (AI) education and data science education for several months now, inviting experts to share their perspectives in a series of very well-attended seminars. At the same time, we’ve been running a programme of research trials to find out what interventions in school might successfully improve gender balance in computing. We’re learning a lot, and one primary lesson is that these topics are not discrete: there are relationships between them.
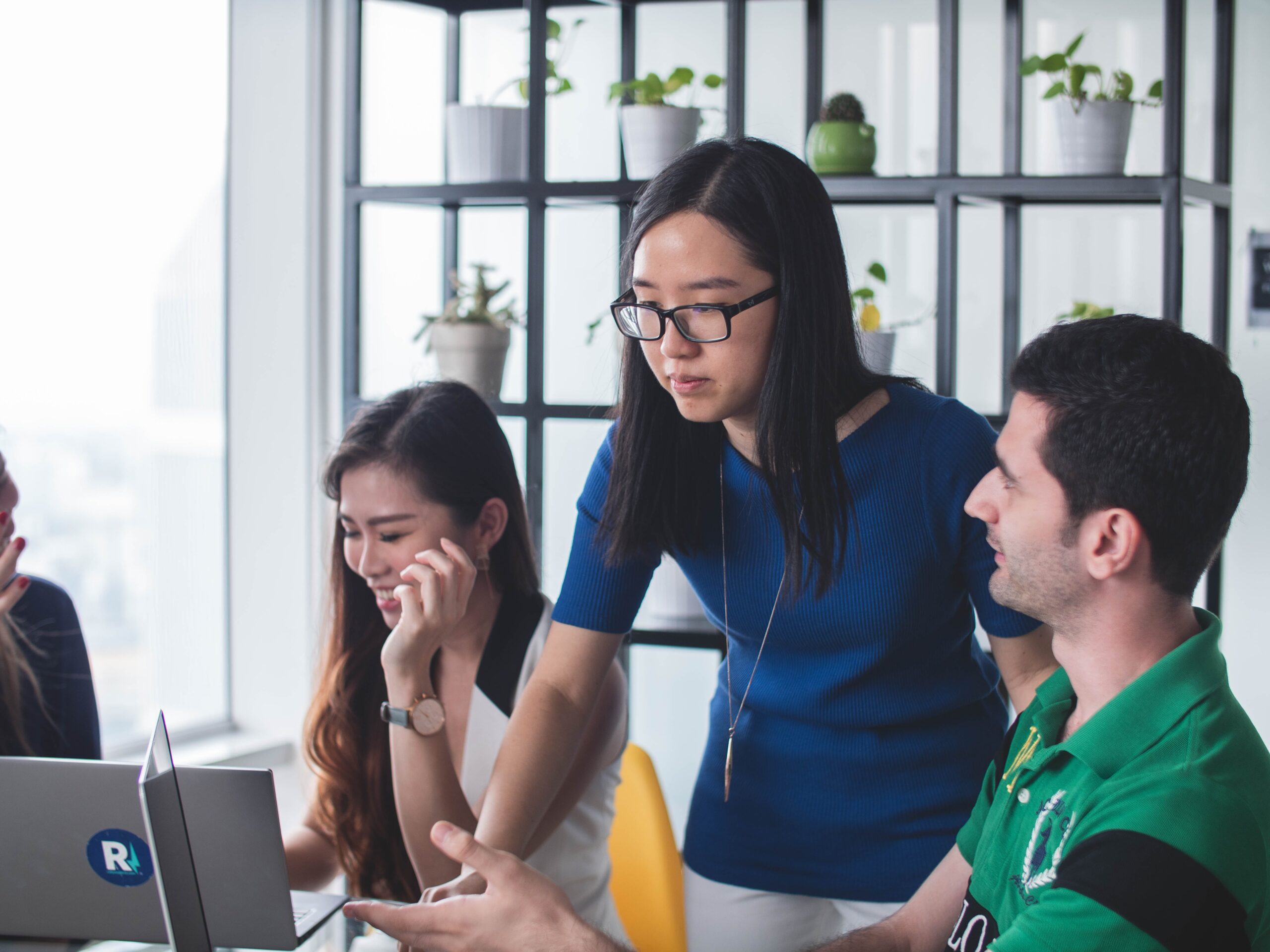
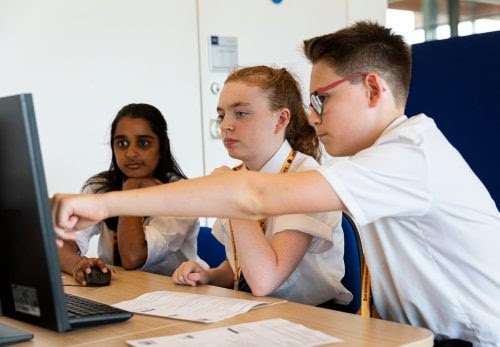
We can’t talk about AI education — or computer science education more generally — without considering the context in which we deliver it, and the societal issues surrounding computing, AI, and data. For this International Women’s Day, I’m writing about the intersection of AI and gender, particularly with respect to gender bias in machine learning.
The quest for gender equality
Gender inequality is everywhere, and researchers, activists, and initiatives, and governments themselves, have struggled since the 1960s to tackle it. As women and girls around the world continue to suffer from discrimination, the United Nations has pledged, in its Sustainable Development Goals, to achieve gender equality and to empower all women and girls.
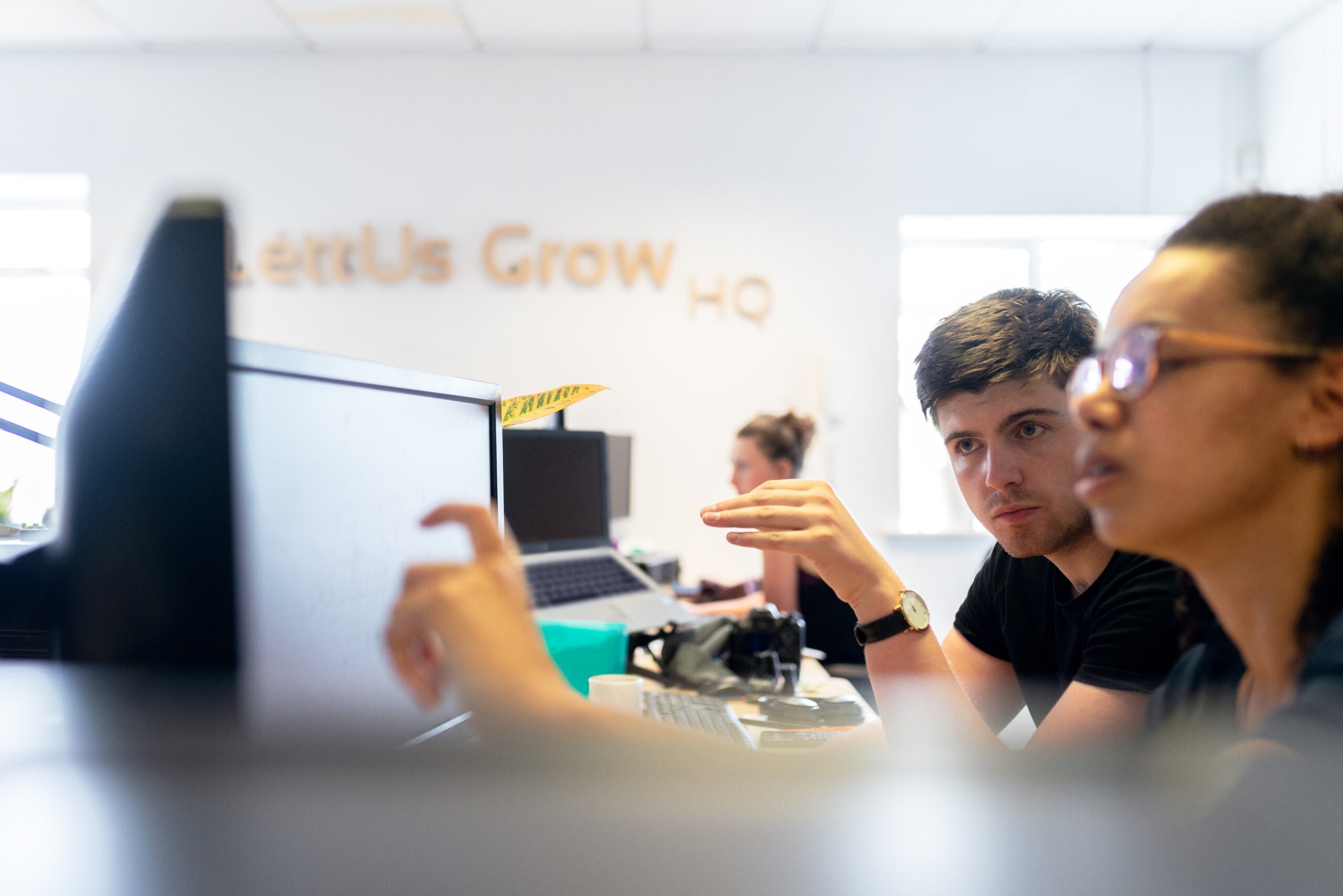
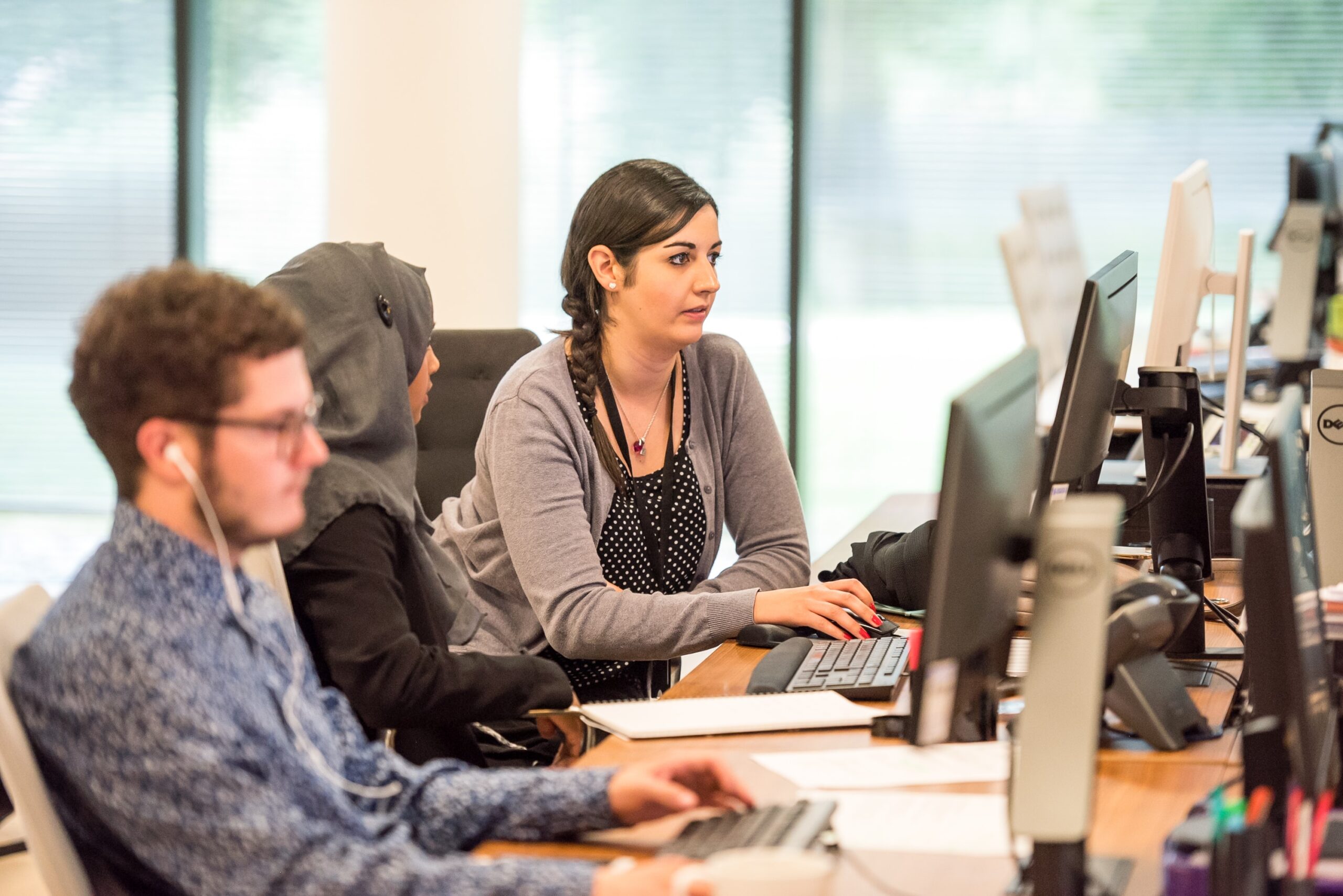
While progress has been made, new developments in technology may be threatening to undo this. As Susan Leavy, a machine learning researcher from the Insight Centre for Data Analytics, puts it:
Artificial intelligence is increasingly influencing the opinions and behaviour of people in everyday life. However, the over-representation of men in the design of these technologies could quietly undo decades of advances in gender equality.
Susan Leavy, 2018 [1]
Gender-biased data
In her 2019 award-winning book Invisible Women: Exploring Data Bias in a World Designed for Men [2], Caroline Criado Perez discusses the effects of gender-biased data. She describes, for example, how the designs of cities, workplaces, smartphones, and even crash test dummies are all based on data gathered from men. She also discusses that medical research has historically been conducted by men, on male bodies.
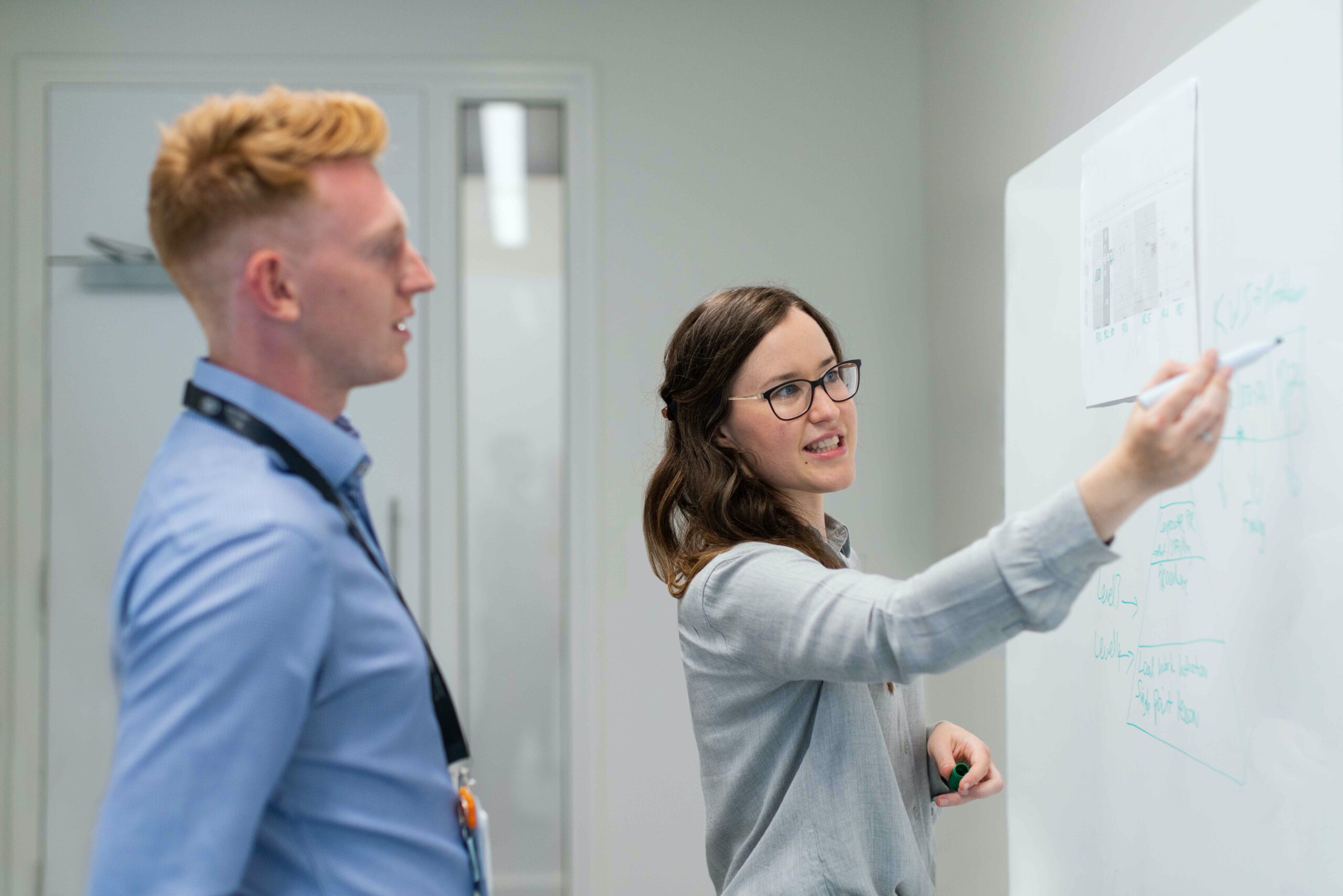
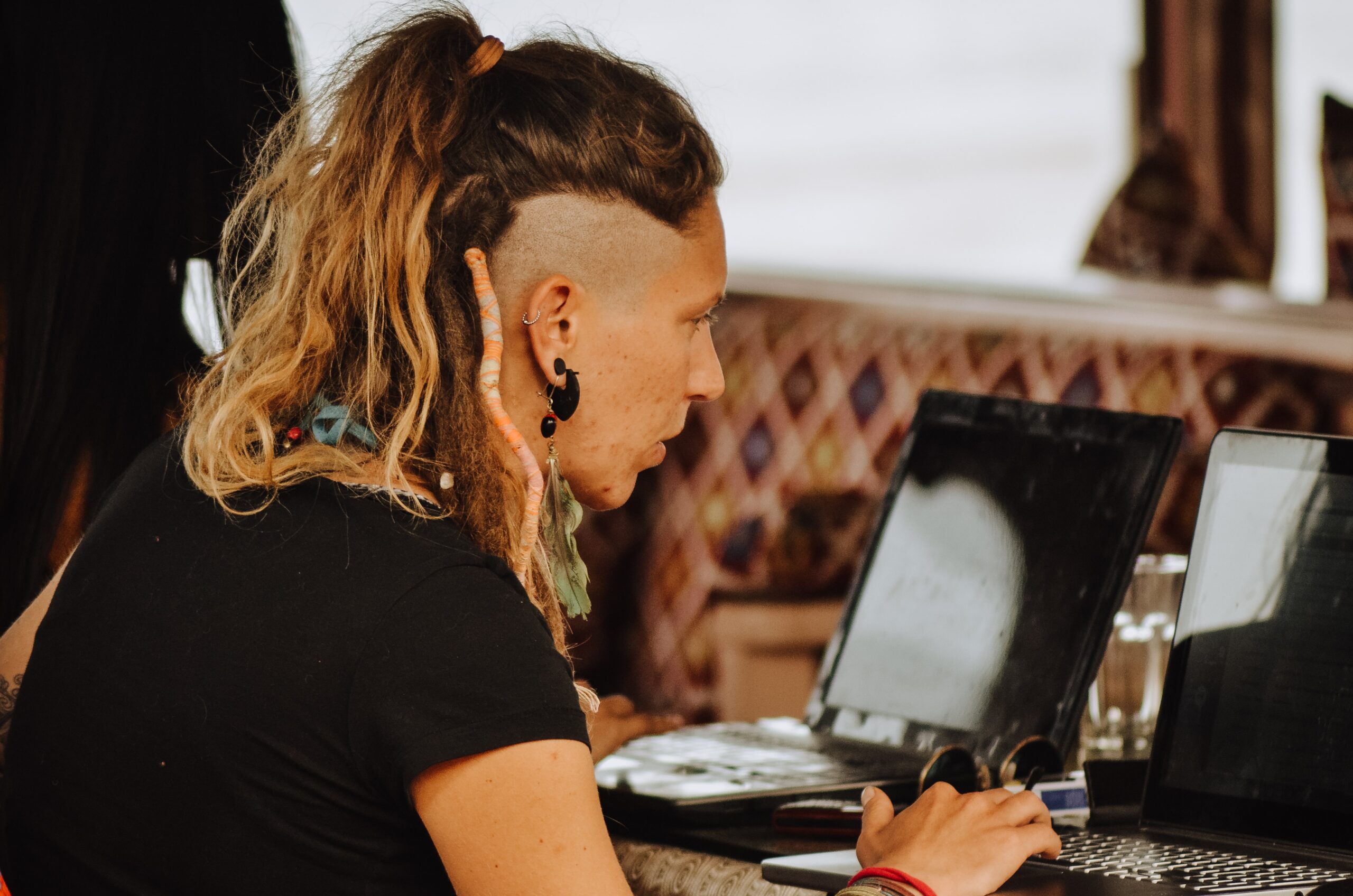
Looking at this problem from a different angle, researcher Mayra Buvinic and her colleagues highlight that in most countries of the world, there are no sources of data that capture the differences between male and female participation in civil society organisations, or in local advisory or decision making bodies [3]. A lack of data about girls and women will surely impact decision making negatively.
Bias in machine learning
Machine learning (ML) is a type of artificial intelligence technology that relies on vast datasets for training. ML is currently being use in various systems for automated decision making. Bias in datasets for training ML models can be caused in several ways. For example, datasets can be biased because they are incomplete or skewed (as is the case in datasets which lack data about women). Another example is that datasets can be biased because of the use of incorrect labels by people who annotate the data. Annotating data is necessary for supervised learning, where machine learning models are trained to categorise data into categories decided upon by people (e.g. pineapples and mangoes).
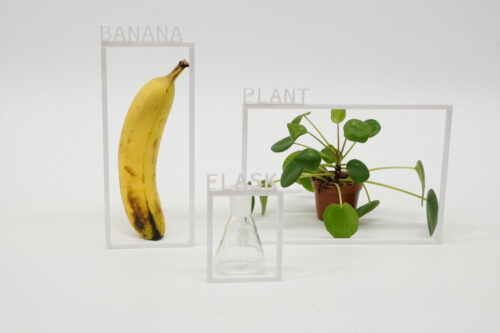
In order for a machine learning model to categorise new data appropriately, it needs to be trained with data that is gathered from everyone, and is, in the case of supervised learning, annotated without bias. Failing to do this creates a biased ML model. Bias has been demonstrated in different types of AI systems that have been released as products. For example:
Facial recognition: AI researcher Joy Buolamwini discovered that existing AI facial recognition systems do not identify dark-skinned and female faces accurately. Her discovery, and her work to push for the first-ever piece of legislation in the USA to govern against bias in the algorithms that impact our lives, is narrated in the 2020 documentary Coded Bias.
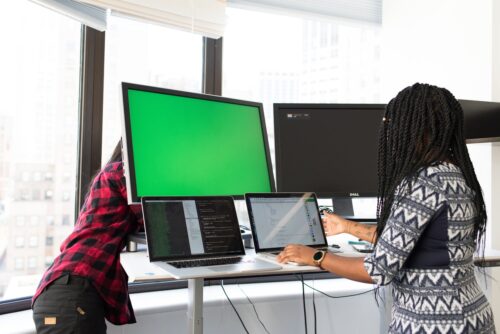
Natural language processing: Imagine an AI system that is tasked with filling in the missing word in “Man is to king as woman is to X” comes up with “queen”. But what if the system completes “Man is to software developer as woman is to X” with “secretary” or some other word that reflects stereotypical views of gender and careers? AI models called word embeddings learn by identifying patterns in huge collections of texts. In addition to the structural patterns of the text language, word embeddings learn human biases expressed in the texts. You can read more about this issue in this Brookings Institute report.
Not noticing
There is much debate about the level of bias in systems using artificial intelligence, and some AI researchers worry that this will cause distrust in machine learning systems. Thus, some scientists are keen to emphasise the breadth of their training data across the genders. However, other researchers point out that despite all good intentions, gender disparities are so entrenched in society that we literally are not aware of all of them. White and male dominance in our society may be so unconsciously prevalent that we don’t notice all its effects.
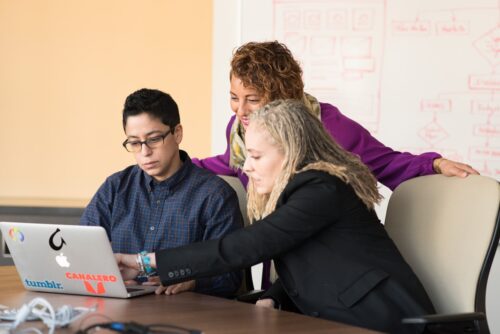
As sociologist Pierre Bourdieu famously asserted in 1977: “What is essential goes without saying because it comes without saying: the tradition is silent, not least about itself as a tradition.” [4]. This view holds that people’s experiences are deeply, or completely, shaped by social conventions, even those conventions that are biased. That means we cannot be sure we have accounted for all disparities when collecting data.
What is being done in the AI sector to address bias?
Developers and researchers of AI systems have been trying to establish rules for how to avoid bias in AI models. An example rule set is given in an article in the Harvard Business Review, which describes the fact that speech recognition systems originally performed poorly for female speakers as opposed to male ones, because systems analysed and modelled speech for taller speakers with longer vocal cords and lower-pitched voices (typically men).
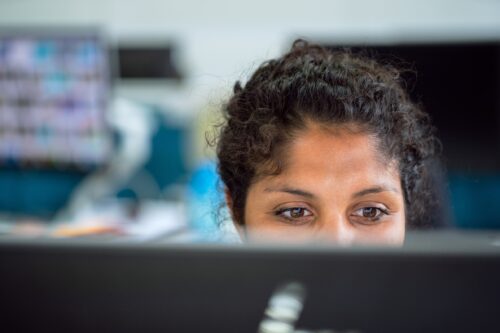
The article recommends four ways for people who work in machine learning to try to avoid gender bias:
- Ensure diversity in the training data (in the example from the article, including as many female audio samples as male ones)
- Ensure that a diverse group of people labels the training data
- Measure the accuracy of a ML model separately for different demographic categories to check whether the model is biased against some demographic categories
- Establish techniques to encourage ML models towards unbiased results
What can everybody else do?
The above points can help people in the AI industry, which is of course important — but what about the rest of us? It’s important to raise awareness of the issues around gender data bias and AI lest we find out too late that we are reintroducing gender inequalities we have fought so hard to remove. Awareness is a good start, and some other suggestions, drawn out from others’ work in this area are:
Improve the gender balance in the AI workforce
Having more women in AI and data science, particularly in both technical and leadership roles, will help to reduce gender bias. A 2020 report by the World Economic Forum (WEF) on gender parity found that women account for only 26% of data and AI positions in the workforce. The WEF suggests five ways in which the AI workforce gender balance could be addressed:
- Support STEM education
- Showcase female AI trailblazers
- Mentor women for leadership roles
- Create equal opportunities
- Ensure a gender-equal reward system
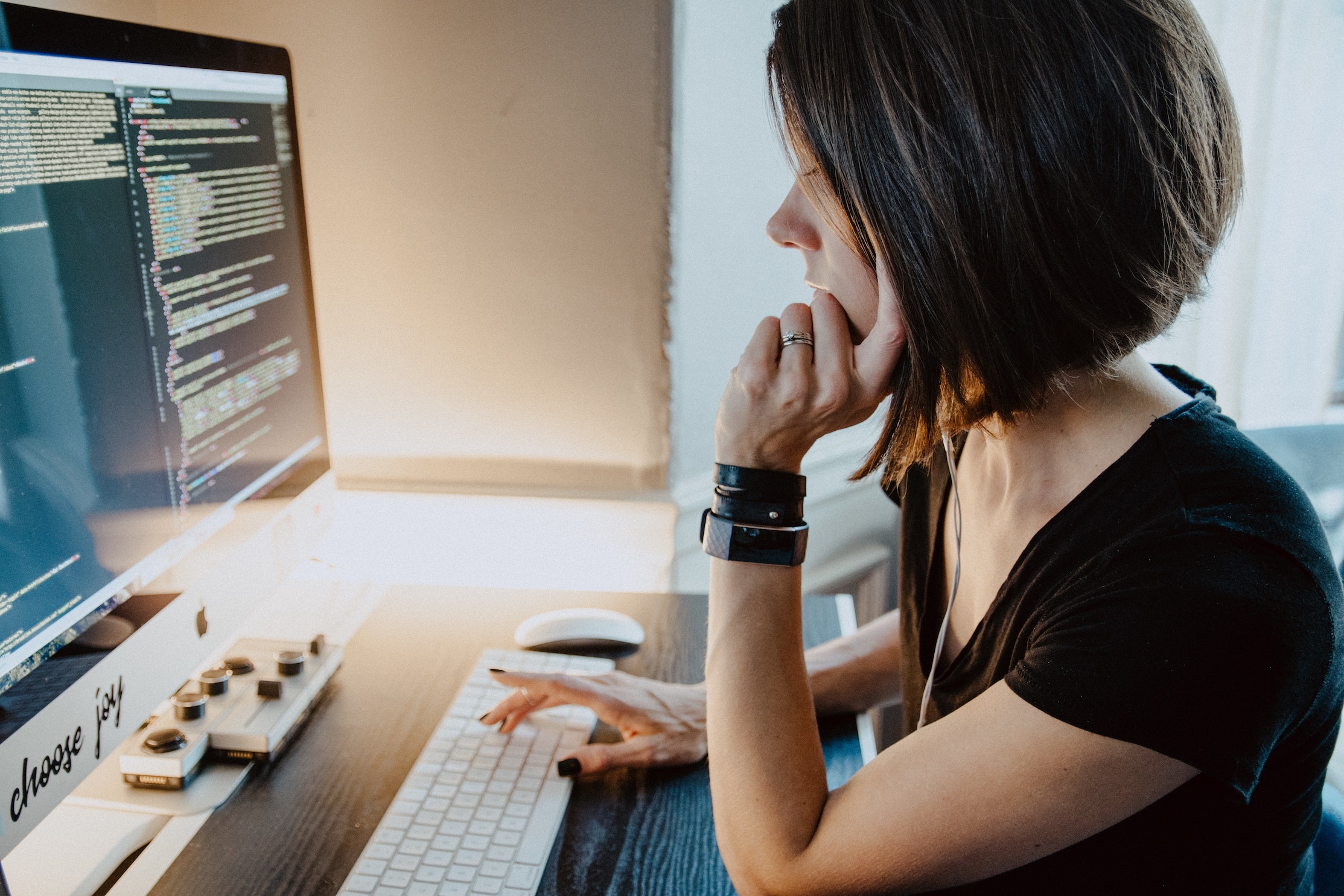
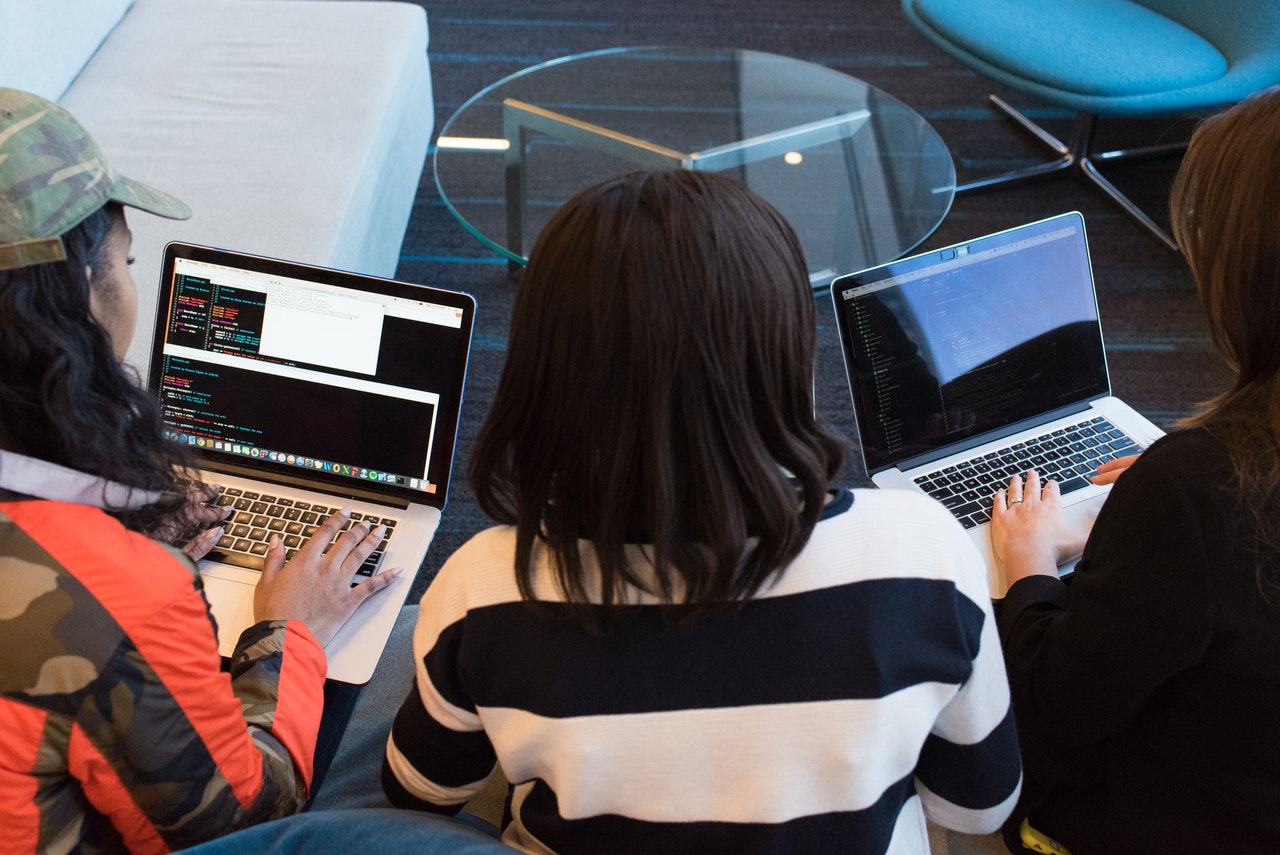
Ensure the collection of and access to high-quality and up-to-date gender data
We need high-quality dataset on women and girls, with good coverage, including country coverage. Data needs to be comparable across countries in terms of concepts, definitions, and measures. Data should have both complexity and granularity, so it can be cross-tabulated and disaggregated, following the recommendations from the Data2x project on mapping gender data gaps.
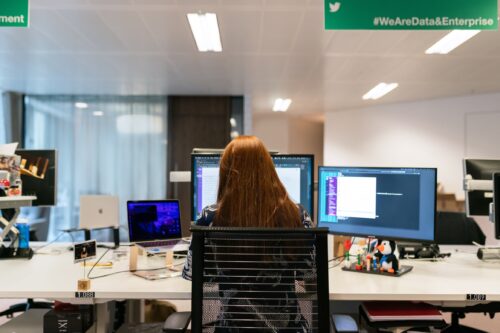
Educate young people about AI
At the Raspberry Pi Foundation we believe that introducing some of the potential (positive and negative) impacts of AI systems to young people through their school education may help to build awareness and understanding at a young age. The jury is out on what exactly to teach in AI education, and how to teach it. But we think educating young people about new and future technologies can help them to see AI-related work opportunities as being open to all, and to develop critical and ethical thinking.
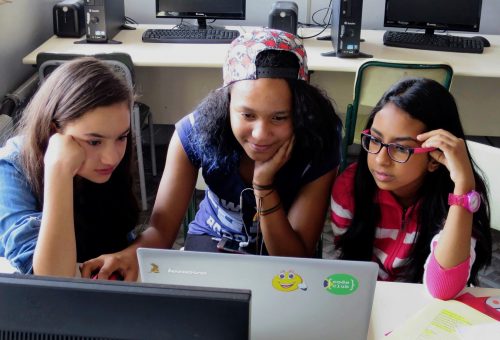
In our AI education seminars we heard a number of perspectives on this topic, and you can revisit the videos, presentation slides, and blog posts. We’ve also been curating a list of resources that can help to further AI education — although there is a long way to go until we understand this area fully.
We’d love to hear your thoughts on this topic.
References
[1] Leavy, S. (2018). Gender bias in artificial intelligence: The need for diversity and gender theory in machine learning. Proceedings of the 1st International Workshop on Gender Equality in Software Engineering, 14–16.
[2] Perez, C. C. (2019). Invisible Women: Exploring Data Bias in a World Designed for Men. Random House.
[3] Buvinic M., Levine R. (2016). Closing the gender data gap. Significance 13(2):34–37
[4] Bourdieu, P. (1977). Outline of a Theory of Practice (No. 16). Cambridge University Press. (p.167)
7 comments
Al Stevens
Still haven’t managed to get a single girl coming along to my new school ‘code club’, using the RPi 400. Do we need a different name, or something much more? 15 enthusiastic boys, zero girls, in a school with mostly-female teachers. Some things never seem to change, sadly.
Raspberry Pi Staff Katharine Childs
Hi Al, great to hear that you’ve got a code club running at your school! Girls are generally well represented in our network of informal learning activities such as Code Clubs and CoderDojos, so there is definitely potential to increase the engagement of girls at your club. Three ideas to consider are:
1) encourage collaborative working such as paired programming, as research suggests girls value opportunities to interact with others with solving problems
2) have your club members work on projects with real-world applications that help to solve problems that are relevant to their lives and communities. Our projects paths are a great place to start: https://projects.raspberrypi.org/en/paths
3) look for opportunities to make sure girls and women are represented in computing at your school. This could be through teachers role modelling confidence in computing, using classroom displays to show a diverse group of people who work in computing careers or by encouraging older students to mentor younger ones.
Al Stevens
Thanks Katharine – it’s early days so we will keep working on this, and try to broaden engagement for next term / next year. Ironically, the main IT teacher is also my son’s class teacher, but she has sadly been off with long covid since October. So the (male) deputy head is helping out instead. We will get there!
Harry Hardjono
I suggest trying out the steAm approach, instead of STEM. Why to code, is a much more important question than How to code. It provides motivation.
To balance gender, you must first identify the differences. Unfortunately, people who took such a step tend to suffer greatly, such as James Damore.
The picture on my March 7, 2022 blogspot on URL above is done by Super Awesome Sylvia’s Watercolor Bot by Evil Mad Scientist and Raspberry Pi. That is what exciting, instead of wrangling SVG on Inkscape, the software I use to create the picture.
Harry Hardjono
URL didn’t take? ramstrong.blogspot.com. The latest projects are done by coding on Raspberry Pi. I also have simpletongeek.blogspot.com, but that one is very technical as I show actual code with little explanations. I doubt many people would be interested. No motivation.
Salomey Afua Addo
Very informative post! Thank you Dr Sue Sentance.
Marina Teramond
Without any doubts, it is so cool that you covered this topic of gender bias in AI because, unfortunately, it is really widespread in our world and a lot of women face it, but we need to strive for eradicating this discrimination. You are absolutely right that gender inequality is everywhere despite huge and long struggle with this. Of course, it is so cool that developers and researchers of AI systems have been trying to establish rules for how to avoid bias in AI models and that there are certain measures which can help to change this situation, but I think that it is important to eradicate the problem of gender bias in AL on a global level. I think that it is important to be partial to this theme and expand people’s consciousness, giving them a huge awareness of gender bias in AL because it requires more attention. Also, creating equal opportunities at workplaces will be a great step for getting rid of gender bias in AL and it needs to be of paramount importance for employers.