How anthropomorphism hinders AI education
In the 1950s, Alan Turing explored the central question of artificial intelligence (AI). He thought that the original question, “Can machines think?”, would not provide useful answers because the terms “machine” and “think” are hard to define. Instead, he proposed changing the question to something more provable: “Can a computer imitate intelligent behaviour well enough to convince someone they are talking to a human?” This is commonly referred to as the Turing test.
It’s been hard to miss the newest generation of AI chatbots that companies have released over the last year. News articles and stories about them seem to be everywhere at the moment. So you may have heard of machine learning (ML) chatbot applications such as ChatGPT and LaMDA. These applications are advanced enough to have caused renewed discussions about the Turing Test and whether the chatbot applications are sentient.
Chatbots are not sentient
Without any knowledge of how people create such chatbot applications, it’s easy to imagine how someone might develop an incorrect mental model around these applications being living entities. With some awareness of Sci-Fi stories, you might even start to imagine what they could look like or associate a gender with them.
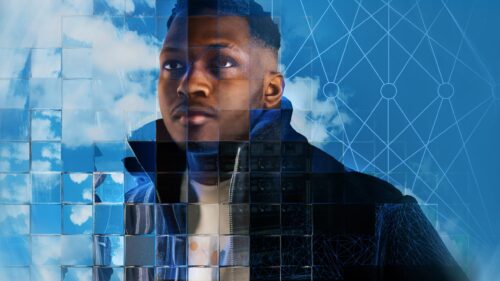
The reality is that these new chatbots are applications based on a large language model (LLM) — a type of machine learning model that has been trained with huge quantities of text, written by people and taken from places such as books and the internet, e.g. social media posts. An LLM predicts the probable order of combinations of words, a bit like the autocomplete function of a smartphone. Based on these probabilities, it can produce text outputs. LLM chatbot applications run on servers with huge amounts of computing power that people have built in data centres around the world.
Our AI education resources for young people
AI applications are often described as “black boxes” or “closed boxes”: they may be relatively easy to use, but it’s not as easy to understand how they work. We believe that it’s fundamentally important to help everyone, especially young people, to understand the potential of AI technologies and to open these closed boxes to understand how they actually work.
As always, we want to demystify digital technology for young people, to empower them to be thoughtful creators of technology and to make informed choices about how they engage with technology — rather than just being passive consumers.
That’s the goal we have in mind as we’re working on lesson resources to help teachers and other educators introduce KS3 students (ages 11 to 14) to AI and ML. We will release these Experience AI lessons very soon.
Why we avoid describing AI as human-like
Our researchers at the Raspberry Pi Computing Education Research Centre have started investigating the topic of AI and ML, including thinking deeply about how AI and ML applications are described to educators and learners.
To support learners to form accurate mental models of AI and ML, we believe it is important to avoid using words that can lead to learners developing misconceptions around machines being human-like in their abilities. That’s why ‘anthropomorphism’ is a term that comes up regularly in our conversations about the Experience AI lessons we are developing.
To anthropomorphise: “to show or treat an animal, god, or object as if it is human in appearance, character, or behaviour”
https://dictionary.cambridge.org/dictionary/english/anthropomorphize
Anthropomorphising AI in teaching materials might lead to learners believing that there is sentience or intention within AI applications. That misconception would distract learners from the fact that it is people who design AI applications and decide how they are used. It also risks reducing learners’ desire to take an active role in understanding AI applications, and in the design of future applications.
Examples of how anthropomorphism is misleading
Avoiding anthropomorphism helps young people to open the closed box of AI applications. Take the example of a smart speaker. It’s easy to describe a smart speaker’s functionality in anthropomorphic terms such as “it listens” or “it understands”. However, we think it’s more accurate and empowering to explain smart speakers as systems developed by people to process sound and carry out specific tasks. Rather than telling young people that a smart speaker “listens” and “understands”, it’s more accurate to say that the speaker receives input, processes the data, and produces an output. This language helps to distinguish how the device actually works from the illusion of a persona the speaker’s voice might conjure for learners.
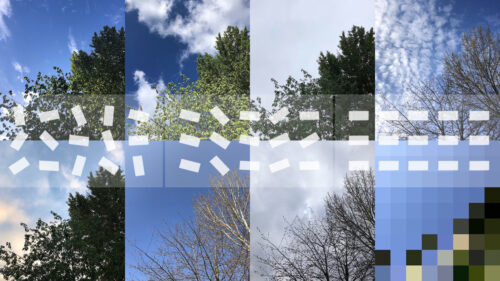
Another example is the use of AI in computer vision. ML models can, for example, be trained to identify when there is a dog or a cat in an image. An accurate ML model, on the surface, displays human-like behaviour. However, the model operates very differently to how a human might identify animals in images. Where humans would point to features such as whiskers and ear shapes, ML models process pixels in images to make predictions based on probabilities.
Better ways to describe AI
The Experience AI lesson resources we are developing introduce students to AI applications and teach them about the ML models that are used to power them. We have put a lot of work into thinking about the language we use in the lessons and the impact it might have on the emerging mental models of the young people (and their teachers) who will be engaging with our resources.
It’s not easy to avoid anthropomorphism while talking about AI, especially considering the industry standard language in the area: artificial intelligence, machine learning, computer vision, to name but a few examples. At the Foundation, we are still training ourselves not to anthropomorphise AI, and we take a little bit of pleasure in picking each other up on the odd slip-up.
Here are some suggestions to help you describe AI better:
Avoid using | Instead use |
Avoid using phrases such as “AI learns” or “AI/ML does” | Use phrases such as “AI applications are designed to…” or “AI developers build applications that…” |
Avoid words that describe the behaviour of people (e.g. see, look, recognise, create, make) | Use system type words (e.g. detect, input, pattern match, generate, produce) |
Avoid using AI/ML as a countable noun, e.g. “new artificial intelligences emerged in 2022” | Refer to ‘AI/ML’ as a scientific discipline, similarly to how you use the term “biology” |
The purpose of our AI education resources
If we are correct in our approach, then whether or not the young people who engage in Experience AI grow up to become AI developers, we will have helped them to become discerning users of AI technologies and to be more likely to see such products for what they are: data-driven applications and not sentient machines.
If you’d like to get involved with Experience AI and use our lessons with your class, you can start by visiting us at experience-ai.org.
5 comments
Mickey
No comments yet on this blog? I read it some time ago and had some thoughts, in agreement.
The anthropic principle applied to physics and cosmology is an embarrassment to modern scientific thought. The same applies to the technology of AI. Artificial Intelligence, as it exists today, comes nowhere near human thought. It currently resembles those few people who are able to do extraordinary feats of memory, computation, musical performance, visual arts, and other activities without the benefit of education or training at its best. This “Rain Man” phenomenon that was known by the unfortunate and unkind epithet of “idiot savant” occurs spontaneously in humans. In AI it requires massive amounts of data fed into a network as “training”.
The AIs in actual use today are totally influenced by the choice of data, which brings us back to an early concept of computing, GIGO, Garbage In Garbage Out. So when, for instance, an AI is trained with existing historical records of people who are eligible for bank loans, it will continue to discriminate against certain disinfranchised people who have been historically discriminated against. Of course the same negative results contaminate law enforcement, employment, rent taking, and other activities previously “legally” and “socially” designed to keep “those people” in “their place”.
Another harmful effect of AI is the confabulation of text that results in conspiracy theories being accepted by some people. This obviously results in political extremism and violence. In these situations, AI could be said to stand for Artificial Ignorance.
Raspberry Pi Staff Ben Garside — post author
You’ve illustrated your points with some great examples of how bias predictions can be a consequence of the bias data used to train ML models.
With the recent emergence of publicly accessible applications that use large language models, such as ChatGPT, it’s not hard to imagine that, without education, many people won’t question their accuracy and will trust their output.
Dave
At the core of AI / ML is the “science” of Mathematics, specifically the fields of Statistics and Probability, and perhaps this is the issue, poor math literacy, which requires the anthropomorphising of these concepts. Hopefully any resources developed would include the mathematical concepts or tightly integrate with the math curricula.
Raspberry Pi Staff Jan Ander
That’s an interesting point, Dave. I also imagine that understanding something about statistics and probability will help people grasp AI/ML concepts more easily. Notably however, the people who first worked on AI, who had very strong math literacy, engaged in anthropomorphism from the start — hence the name artificial intelligence, and the founding question “Can machines think?”. And this trend continued as the field developed (e.g. adding terms like machine learning, neural networks). So understanding statistics and probability clearly doesn’t prevent people from anthropomorphising computing technologies.
You might be interested in Conrad Wolfram’s work on developing a mathematics curriculum “for the AI age” — he spoke at our seminar series last October.
Jason Stathum
Great post! Your exploration of anthropomorphism’s impact on AI education is insightful and well-presented. It’s essential to demystify AI for young learners and provide them with accurate mental models. Your efforts to avoid anthropomorphism in AI descriptions are commendable and align with our shared goal of promoting understanding and informed engagement with technology. I’ll definitely check out your blog for more valuable information on AI education. Keep up the fantastic work! Please visit my blog as well for similar discussions on AI topics. Let’s continue spreading knowledge and awareness about AI together!