How can AI-based analysis help educators support students?
We are hosting a series of free research seminars about how to teach artificial intelligence (AI) and data science to young people, in partnership with The Alan Turing Institute.
In the fifth seminar of this series, we heard from Rose Luckin, Professor of Learner Centred Design at the University College London (UCL) Knowledge Lab. Rose is Founder of EDUCATE Ventures Research Ltd., a London consultancy service working with start-ups, researchers, and educators to develop evidence-based educational technology.
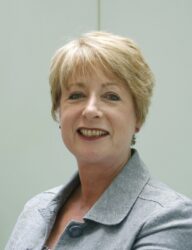
Based on her experience at EDUCATE, Rose spoke about how AI-based analysis could help educators gain a deeper understanding of their students, and how educators could work with AI systems to provide better learning resources to their students. This provided us with a different angle to the first four seminars in our current series, where we’ve been thinking about how young people learn to understand AI systems.
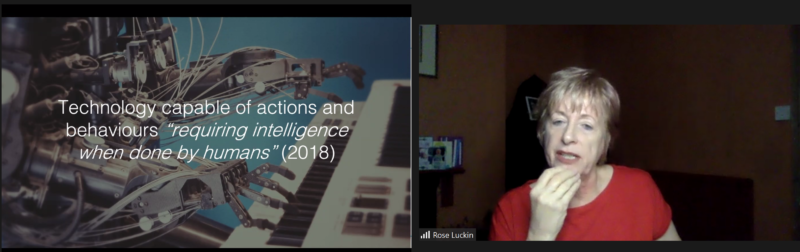
Education and AI systems
AI systems have the potential to impact education in a number of different ways, which Rose distilled into three areas:
- Using AI in education to tackle some of the big educational challenges
- Educating teachers about AI so that they can use it safely and effectively
- Changing education so that we focus on human intelligence and prepare people for an AI world
It is clear that the three areas are interconnected, meaning developments in one area will affect the others. Rose’s focus during the seminar was the second area: educating people about AI.
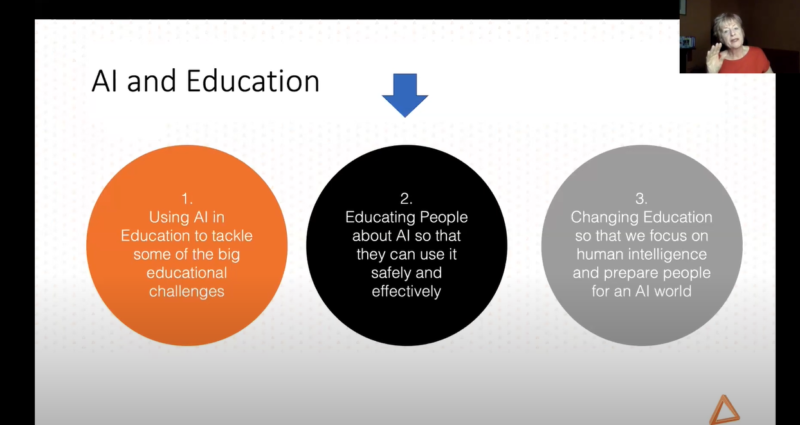
What can AI systems do in education?
Through giving examples of existing AI-based systems used for education, Rose described what in particular it is about AI systems that can be useful in an education setting. The first point she raised was that AI systems can adapt based on learning from data. Her main example was the AI-based platform ENSKILLS, which detects the user’s level of competency with spoken English through the user’s interactions with a virtual character, and gradually adapts the character to the user’s level. Other examples of adaptive AI systems for education include Carnegie Learning and Century Intelligent Learning.
We know that AI systems can respond to different forms of data. Rose introduced the example of OyaLabs to demonstrate how AI systems can gather and process real-time sensory data. This is an app that parents can use in a young child’s room to monitor the child’s interactions with others. The app analyses the data it gathers and produces advice for parents on how they can support their child’s language development.
AI system creators can also combine adaptivity and real-time sensory data processing in their systems. One example Rosa gave of this was SimSensei from the University of Southern California. This is a simulated coach, which a student can interact with and which gathers real-time data about how the student is speaking, including their tone, speed of speech, and facial expressions. The system adapts its coaching advice based on these interactions and on what it learns from interactions with other students.
Getting ready for AI systems in education
For the remainder of her presentation, Rose focused on the framework she is involved in developing, as part of the EDUCATE service, to support organisations to prepare for implementing AI systems, including educators within these organisations. The aim of this ETHICAI framework is to enable organisations and educators to understand:
- What AI systems are capable of doing
- The strengths and weaknesses of AI systems
- How data is used by AI systems to learn
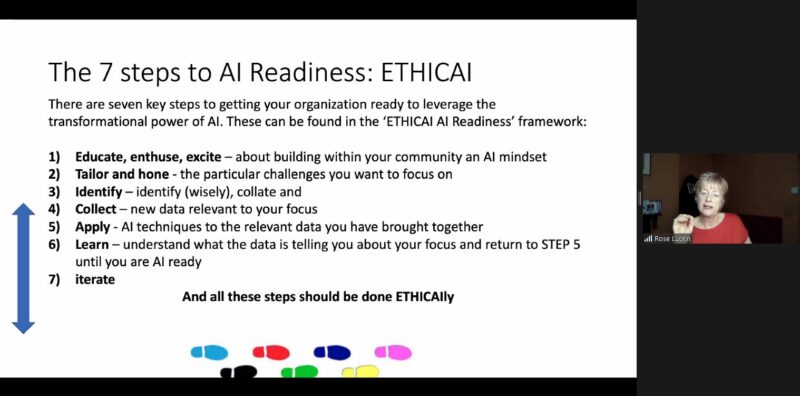
Rose described the seven steps of the framework as:
- Educate, enthuse, excite – about building an AI mindset within your community
- Tailor and Hone – the particular challenges you want to focus on
- Identify – identify (wisely), collate and …
- Collect – new data relevant to your focus
- Apply – AI techniques to the relevant data you have brought together
- Learn – understand what the data is telling you about your focus and return to step 5 until you are AI ready
- Iterate
She then went on to demonstrate how the framework is applied using the example of online teaching. Online teaching has been a key part of education throughout the coronavirus pandemic; AI systems could be used to analyse datasets generated during online teaching sessions, in order to make decisions for and recommendations to educators.
The first step of the ETHICAI framework is educate, enthuse, excite. In Rose’s example, this step consisted of choosing online teaching as a scenario, because it is very pertinent to a teacher’s practice. The second step is to tailor and hone in on particular challenges that are to be the focus, capitalising on what AI systems can do. In Rose’s example, the challenge is assessing the quality of online lessons in a way that would be useful to educators. The third step of the framework is to identify what data is required to perform this quality assessment.
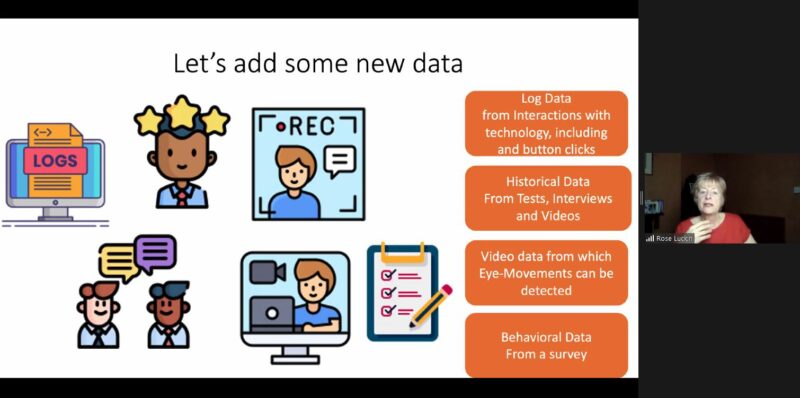
The fourth step is the collection of new data relevant to the focus of the project. The aim is to gain an increased understanding of what happens in online learning across thousands of schools. Walking through the online learning example, Rose suggested we might be able to collect the following types of data:
- Log data
- Audio data
- Performance data
- Video data, which includes eye-movement data
- Historical data from tests and interviews
- Behavioural data from surveying teachers and parents about how they felt about online learning
It is important to consider the ethical implications of gathering all this data about students, something that was a recurrent theme in both Rose’s presentation and the Q&A at the end.
Step five of the ETHICAI framework focuses on applying AI techniques to the relevant data to combine and process it. The figure below shows that in preparation, the various data sets need to be collated, cleaned, organised, and transformed.
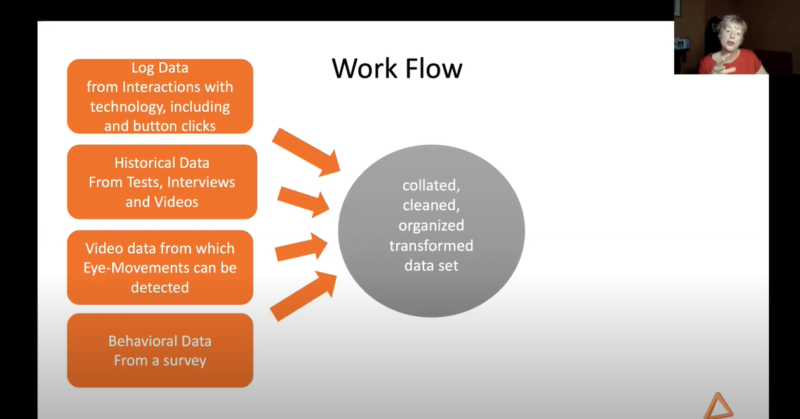
From the correctly prepared data, interaction profiles can be produced in order to put characteristics from different lessons into groups/profiles. Rose described how cluster analysis using a combination of both AI and human intelligence could be used to sort lessons into groups based on common features.
The sixth step in Rose’s example focused on what may be learned from analysing collected data linked to the particular challenge of online teaching and learning. Rose said that applying an AI system to students’ behavioural data could, for example, give indications about students’ focus and confidence, and make or recommend interventions to educators accordingly.
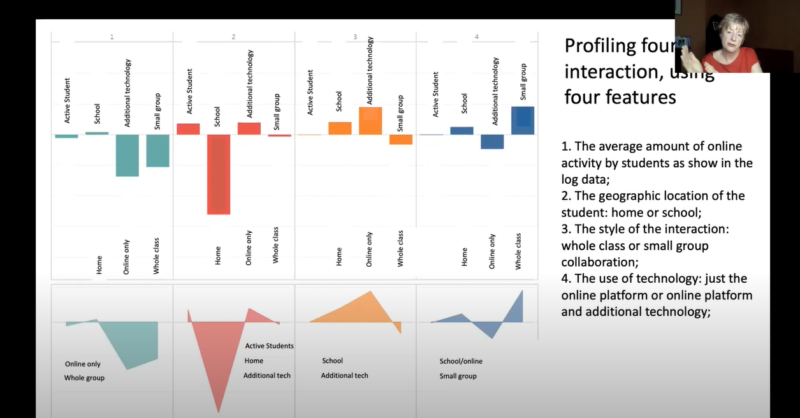
Where might we take applications of AI systems in education in the future?
Rose described that AI systems can possess some types of intelligence humans have or can develop: interdisciplinary academic intelligence, meta-knowing intelligence, and potentially social intelligence. However, there are types such as meta-contextual intelligence and perceived self-efficacy that AI systems are not able to demonstrate in the way humans can.
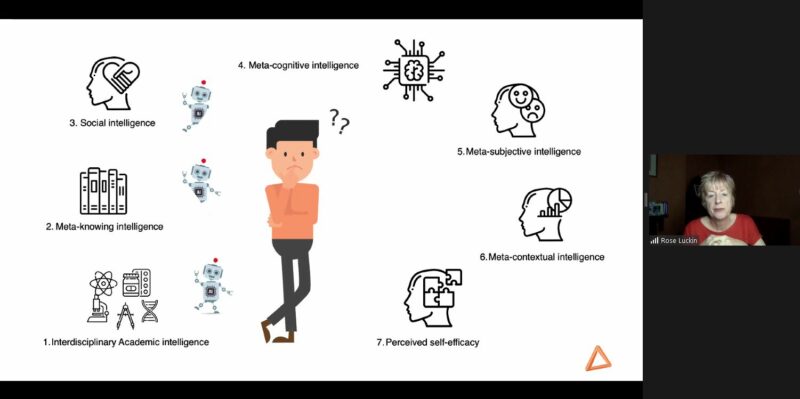
The use of AI systems in education can cause ethical issues. As an example, Rose pointed out the use of virtual glasses to identify when students need help, even if they do not realise it themselves. A system like this could help educators with assessing who in their class needs more help, and could link this back to student performance. However, using such a system like this has obvious ethical implications, and some of these were the focus of the Q&A that followed Rose’s presentation.
It’s clear that, in the education domain as in all other domains, both positive and negative outcomes of integrating AI are possible. In a recent paper written by Wayne Holmes (also from the UCL Knowledge Lab) and co-authors, ‘Ethics of AI in Education: Towards a Community Wide Framework’ [1], the authors suggest that the interpretation of data, consent and privacy, data management, surveillance, and power relations are all ethical issues that should be taken into consideration. Finding consensus for a practical ethical framework or set of principles, with all stakeholders, at the very start of an AI-related project is the only way to ensure ethics are built into the project and the AI system itself from the ground up.
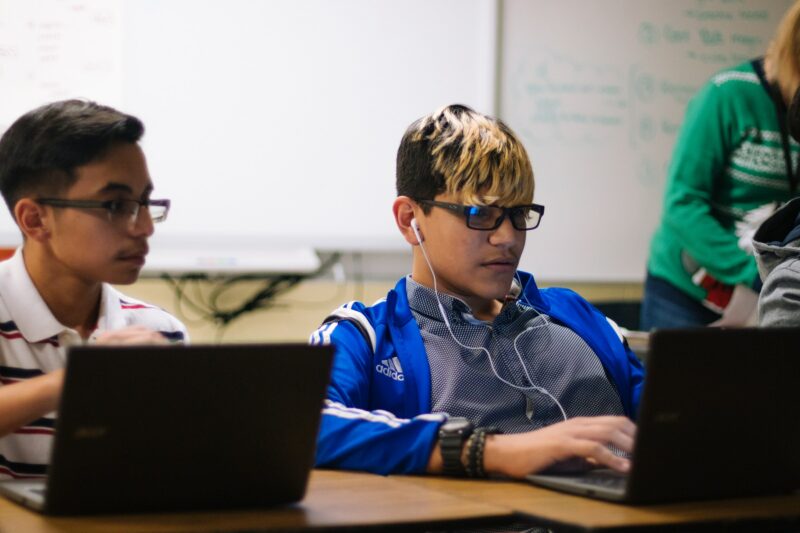
Ethical issues of AI systems more broadly, and how to involve young people in discussions of AI ethics, were the focus of our seminar with Dr Mhairi Aitken back in September. You can revisit the seminar recording, presentation slides, and summary blog post.
I really enjoyed both the focus and content of Rose’s talk: educators understanding how AI systems may be applied to education in order to help them make more informed decisions about how to best support their students. This is an important factor to consider in the context of the bigger picture of what young people should be learning about AI. The work that Rose and her colleagues are doing also makes an important contribution to translating research into practical models that teachers can use.
Join our next free seminars
You may still have time to sign up for our Tuesday 11 January seminar, today at 17:00–18:30 GMT, where we will welcome Dave Touretzky and Fred Martin, founders of the influential AI4K12 framework, which identifies the five big ideas of AI and how they can be integrated into education.
Next month, on 1 February at 17:00–18:30 GMT, Tara Chklovski (CEO of Technovation) will give a presentation called Teaching youth to use AI to tackle the Sustainable Development Goals at our seminar series.
If you want to join any of our seminars, click the button below to sign up and we will send you information on how to join. We look forward to seeing you there!
You’ll always find our schedule of upcoming seminars on this page. For previous seminars, you can visit our past seminars and recordings page.
No comments